LLM(LoRA + LLamaFactory + LLama.cpp + 腾讯cloud studio + 魔塔ModelScope)微调大模型完整流程(一)
六、 启动 LLamaFactory 的 webui,开始微调(参数自行选择或者百度)
1、启动
注意:这里需要服务器开启 7860 端口
llamafactory-cli webui
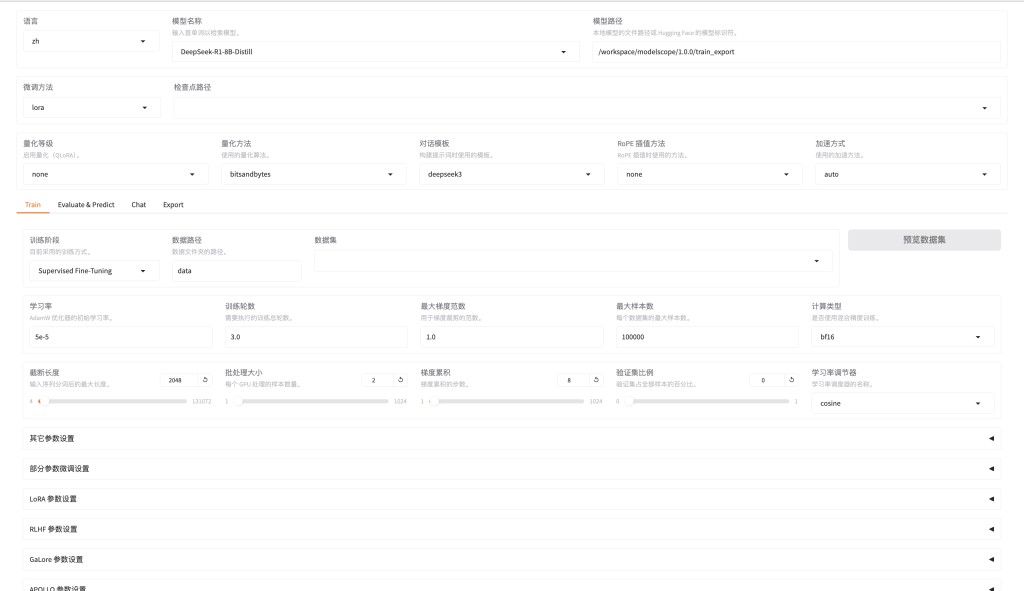
2、微调后,导出模型
CUDA_VISIBLE_DEVICES=0 llamafactory-cli export \
--model_name_or_path /workspace/models/unsloth/DeepSeek-R1-Distill-Llama-8B \ # 这里是预训练模型
--adapter_name_or_path /workspace/saves/DeepSeek-R1-8B-Distill/lora/v101 \ # 这里是微调后的模型
--template deepseek3 \ # 微调时用的模板名称
--finetuning_type lora \
--export_dir /workspace/export/7/v101 \ # 导出目录
--export_size 5 \ # 导出模型大小限制,超过5G就就行分片
--export_device cpu \
--export_legacy_format False
七、转换为 GGUF
cd llama.cpp
1、转换类型
# -outtype f32,f16,bf16,q8_0,tq1_0,tq2_0,auto
2、f16 类型
# python convert_hf_to_gguf.py 导出的模型目录 --split-max-size 超过20G进行分片(这里先用大的没关系,后面量化会压缩大小) --outtype 用f16类型
python convert_hf_to_gguf.py /workspace/export/7/v101 --split-max-size 20G --outtype f16
八、量化
# 帮助命令,可以看到能量化的格式
llama-quantize -h
llama-quantize Train-Export-8.0B-F16.gguf DeepSeek-R1-8B-F16-Q4_K_M.gguf Q4_K_M
# llama-quantize Train-Export-8.0B-F16.gguf DeepSeek-R1-8B-F16-Q4_K_S.gguf Q4_K_S
# llama-quantize Train-Export-8.0B-F16.gguf DeepSeek-R1-8B-F16-Q5_K_M.gguf Q5_K_M
# llama-quantize Train-Export-8.0B-F16.gguf DeepSeek-R1-8B-F16-Q5_K_S.gguf Q5_K_S
# llama-quantize Train-Export-8.0B-F16.gguf DeepSeek-R1-8B-F16-Q6_K.gguf Q6_K
# llama-quantize Train-Export-8.0B-F16.gguf DeepSeek-R1-8B-F16-Q8_0.gguf Q8_0
九、上传到 modelscope(魔塔)
1、初始化git
git init
2、设置仓库
git remote add modelscope https://oauth2:魔塔token@www.modelscope.cn/仓库
3、拉取仓库
git pull modelscope master
4、添加大模型文件格式
git lfs track "*.gguf"
5、添加文件到git仓库
git add .
git commit -m "提交大模型文件和配置文件"
git push modelscope master
附录
这是我微调时用到的参数 config.yaml
top.booster: auto
top.checkpoint_path: []
top.finetuning_type: lora
top.model_name: DeepSeek-R1-8B-Distill
top.quantization_bit: '4'
top.quantization_method: bitsandbytes
top.rope_scaling: linear
top.template: deepseek3
train.additional_target: ''
train.apollo_rank: 16
train.apollo_scale: 32
train.apollo_target: all
train.apollo_update_interval: 200
train.badam_mode: layer
train.badam_switch_interval: 50
train.badam_switch_mode: ascending
train.badam_update_ratio: 0.05
train.batch_size: 4
train.compute_type: bf16
train.create_new_adapter: false
train.cutoff_len: 8192
train.dataset:
- real_estate
train.dataset_dir: /workspace/LLaMA-Factory/data
train.ds_offload: false
train.ds_stage: none
train.extra_args: '{"optim": "adamw_torch"}'
train.freeze_extra_modules: ''
train.freeze_trainable_layers: 2
train.freeze_trainable_modules: all
train.galore_rank: 16
train.galore_scale: 2
train.galore_target: all
train.galore_update_interval: 200
train.gradient_accumulation_steps: 8
train.learning_rate: 5e-5
train.logging_steps: 1
train.lora_alpha: 16
train.lora_dropout: 0.05
train.lora_rank: 16
train.lora_target: ''
train.loraplus_lr_ratio: 24
train.lr_scheduler_type: cosine
train.mask_history: false
train.max_grad_norm: '1.0'
train.max_samples: '517'
train.neat_packing: false
train.neftune_alpha: 0
train.num_train_epochs: '6'
train.packing: false
train.ppo_score_norm: false
train.ppo_whiten_rewards: false
train.pref_beta: 0.1
train.pref_ftx: 0
train.pref_loss: sigmoid
train.report_to:
- none
train.resize_vocab: false
train.reward_model: []
train.save_steps: 10
train.swanlab_api_key: ''
train.swanlab_link: ''
train.swanlab_mode: cloud
train.swanlab_project: llamafactory
train.swanlab_run_name: ''
train.swanlab_workspace: ''
train.train_on_prompt: false
train.training_stage: Supervised Fine-Tuning
train.use_apollo: false
train.use_badam: false
train.use_dora: false
train.use_galore: false
train.use_llama_pro: false
train.use_pissa: false
train.use_rslora: false
train.use_swanlab: false
train.val_size: 0
train.warmup_steps: 20